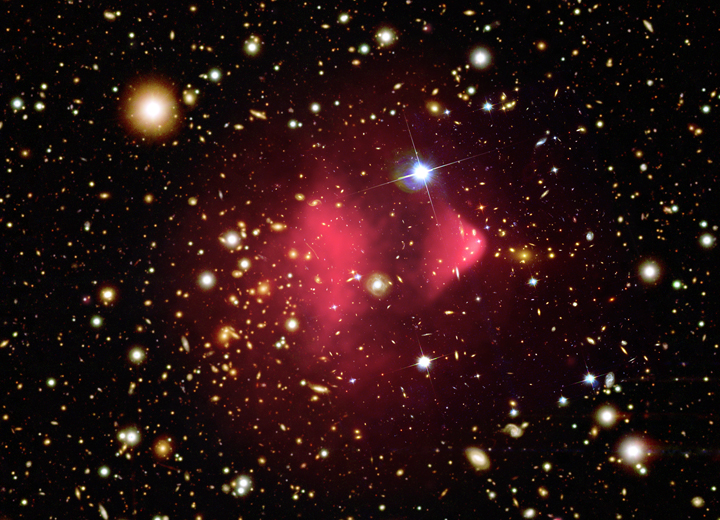
Estimating Galaxy Cluster Mass Accretion Rates (2022-2024)
As the Universe ages, galaxy clusters grow in mass by incorporating neighboring gas, galaxies, and galaxy clusters. This process can visibly disturb the otherwise relaxed galaxy clusters, and biases measurements of galaxy cluster mass. Unfortunately, mass accretion history is very difficult to measure quantitatively. I am recently developed a deep learning model, using simulated cluster observations from the MillenniumTNG simulation, that can estimate the mass accretion rate of a galaxy cluster from X-ray and thermal SZ observations. This model also provides uncertainties for those estimates. Interpretability analysis suggests that the model is using a combination of radial profile information in the cluster and cluster asymmetry.
J. Soltis, M. Ntampaka, B. Diemer, J. ZuHone, S. Bose, A. M. Delgado, B. Hadzhiyska, C. Hernandez-Aguayo, D. Nagai, H. Trac. “A Multi-Wavelength Technique for Estimating Galaxy Cluster Mass Accretion Rates”, arXiv preprint (2024). NASA/ADS link.
Image of combined Optical and X-ray observations of two colliding clusters, including the famous "Bullet Cluster". Credit: X-ray: NASA/CXC/CfA/M.Markevitch et al.; Optical: NASA/STScI; Magellan/U.Arizona/D.Clowe et al.